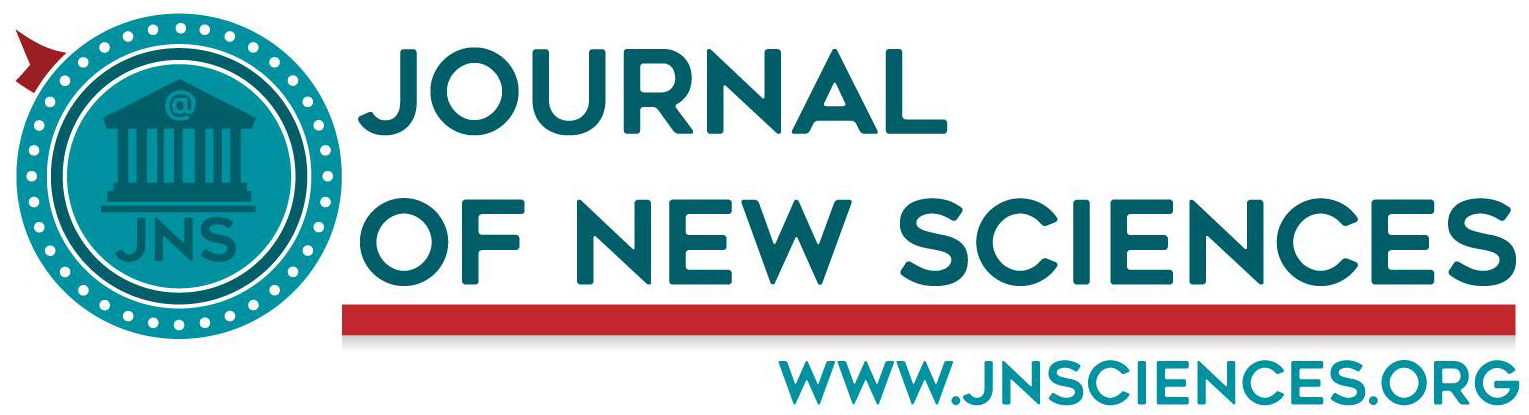
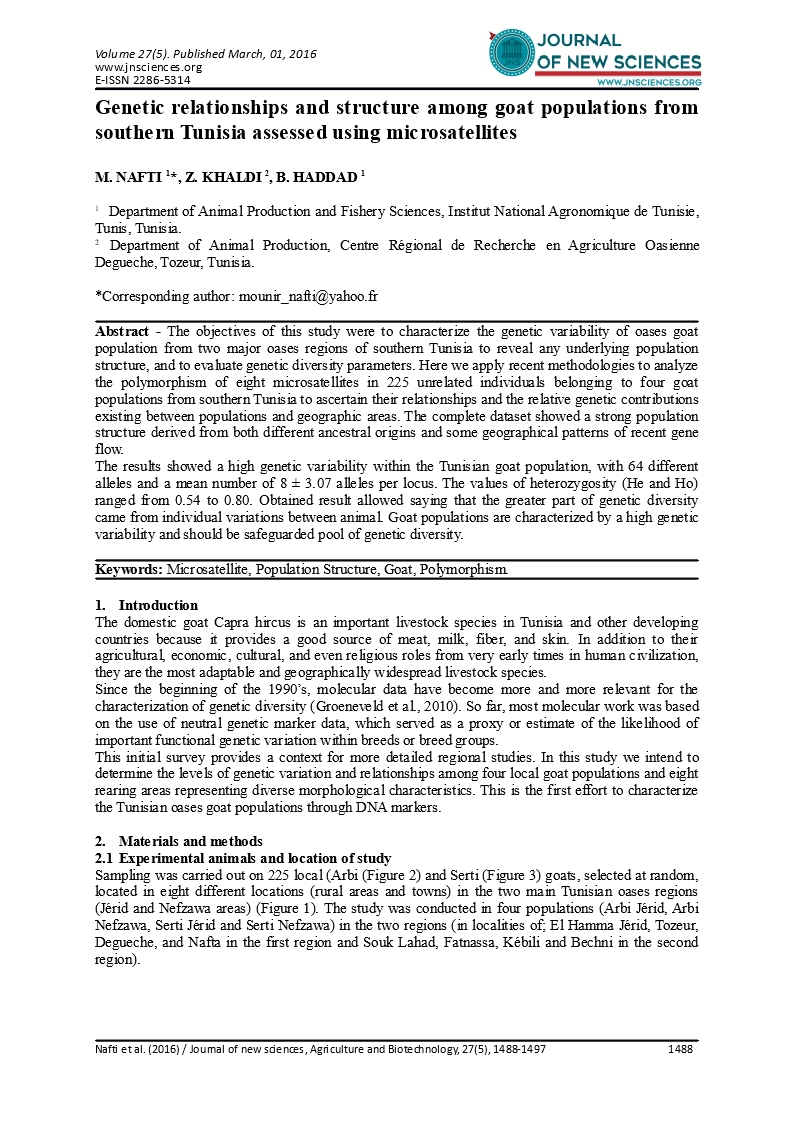
Genetic relationships and structure among goat populations from southern Tunisia assessed using microsatellites
M. Nafti 1*
Z. Khaldi 2
B. Haddad 1
1 Department of Animal Production and Fishery Sciences, Institut National Agronomique de Tunisie, Tunis, Tunisia.
2 Department of Animal Production, Centre Régional de Recherche en Agriculture Oasienne Degueche, Tozeur, Tunisia.
Abstract - The objectives of this study were to characterize the genetic variability of oases goat population from two major oases regions of southern Tunisia to reveal any underlying population structure, and to evaluate genetic diversity parameters. Here we apply recent methodologies to analyze the polymorphism of eight microsatellites in 225 unrelated individuals belonging to four goat populations from southern Tunisia to ascertain their relationships and the relative genetic contributions existing between populations and geographic areas. The complete dataset showed a strong population structure derived from both different ancestral origins and some geographical patterns of recent gene flow.
The results showed a high genetic variability within the Tunisian goat population, with 64 different alleles and a mean number of 8 ± 3.07 alleles per locus. The values of heterozygosity (He and Ho) ranged from 0.54 to 0.80. Obtained result allowed saying that the greater part of genetic diversity came from individual variations between animal. Goat populations are characterized by a high genetic variability and should be safeguarded pool of genetic diversity.
Keywords: Microsatellite, Population Structure, Goat, Polymorphism.
-
Introduction
The domestic goat Capra hircus is an important livestock species in Tunisia and other developing countries because it provides a good source of meat, milk, fiber, and skin. In addition to their agricultural, economic, cultural, and even religious roles from very early times in human civilization, they are the most adaptable and geographically widespread livestock species.
Since the beginning of the 1990’s, molecular data have become more and more relevant for the characterization of genetic diversity (Groeneveld et al., 2010). So far, most molecular work was based on the use of neutral genetic marker data, which served as a proxy or estimate of the likelihood of important functional genetic variation within breeds or breed groups.
This initial survey provides a context for more detailed regional studies. In this study we intend to determine the levels of genetic variation and relationships among four local goat populations and eight rearing areas representing diverse morphological characteristics. This is the first effort to characterize the Tunisian oases goat populations through DNA markers.
-
Materials and methods
-
Experimental animals and location of study
Sampling was carried out on 225 local (Arbi (Figure 2) and Serti (Figure 3) goats, selected at random, located in eight different locations (rural areas and towns) in the two main Tunisian oases regions (Jérid and Nefzawa areas) (Figure 1). The study was conducted in four populations (Arbi Jérid, Arbi Nefzawa, Serti Jérid and Serti Nefzawa) in the two regions (in localities of; El Hamma Jérid, Tozeur, Degueche, and Nafta in the first region and Souk Lahad, Fatnassa, Kébili and Bechni in the second region).
|
Figure 1. Study zone |
|
Figure 2. Arbi goat |
|
Figure 3. Serti goat |
-
Experimental populations
The study was conducted in eight localities from the two major oases areas of Tunisia Jerid and Nefzawa. Arbi Jérid and Serti Jérid goats were studied in the Jérid area. Arbi Nezawa and Serti Nefawa goats were studied in the Nefzawa area. The sampled animals showed an important morphological diversity. This genetic diversity was confirmed by (Nafti et al., 2014).
-
Blood sampling and DNA extraction
Whole blood samples were collected 225 does from indigenous goats of the region of south Tunisian oasis. Blood was collected from each animal via jugular venipuncture into EDTA coated Vaccutainer™ tubes and stored at -20ºC until DNA extraction. Total genomic DNA was extracted using Blood DNA Preparation kit (Jena Biosciences GmbH, Germany) following the manufacturer’s protocol.
-
Microsatellite Markers
Most genetic characterization studies have analyzed highly polymorphic microsatellite markers, which are repeated sequences of 1-4 base pairs. Variability is in terms of the number of repeated sequences observed. Microsatellites do not encode proteins and are thus assumed to be selectively neutral. Microsatellites are now available for most livestock species and have proven their value for studying variation within and across breeds.
The primer pairs used for selective amplification of different microsatellite markers were synthesized by Operon Technologies, Alameda, CA (Table 1).
Table 1. Characteristics of the 8 microsatellite loci used to characterize 225 indigenous Tunisian goats
Primer |
Chromosome Number |
Size Range |
Sequence (forward + reverse) |
|||
TGLA057 |
1 |
122-164 |
F 5’-GCT TTT TAA TCC TCA GCT TGC TG-3’ F 5’-GCT TCC AAA ACT TTA CAA TAT GTA T-3’ |
|||
OarFCB20 |
2 |
99 - 125 |
F 5’-AAA TGT GTT TAA GAT TCC ATA CAG TG-3’ R 5’-GGA AAA CCC CCA TAT ATA CCT ATA C-3’ |
|||
MCM527 |
5 |
155 - 173 |
F 5’-GTC CAT TGC CTC AAA TCA ATT C-3’ R 5’-AAA CCA CTT GAC TAC TCC CCA A-3’ |
|||
RM004 |
15 |
138 - 146 |
F 5’-CAG CAA AAT ATC AGC AAA CCT-3’ R 5’-CCA CCT GGG AAG GCC TTT A-3’ |
|||
MAF65 |
15 |
117 - 127 |
F 5’-AAA GGC CAG AGT ATG CAA TTA GGA G-3’ R 5’-CCA CTC CTC TGA GAA TAT AAC ATG-3’ |
|||
MAF214 |
16 |
174 - 282 |
F 5’-GGG TGA TCT TAG GGA GGT TTT GGA GG-3’ F 5’-AAT GCA GGA GAT CTG AGG CAG GGA CG-3’ |
|||
CSSM31 |
23 |
80-104 |
F 5’-CCA AGT TTA GTA CTT GTA AGT AGA-3’ F 5’-GAC TCT CTA GCA CTT TAT CTG TGT-3’ |
|||
ILSTS087 |
28 |
145 - 165 |
F 5’-AGC AGA CAT GAT GAC TCA GC-3’ R 5’-CTG CCT CTT TTC TTG AGA GC-3’ |
-
Microsatellite loci amplification and genotyping
Selective amplification of different microsatellite markers was performed using BioRad Thermal cycler T100 (Applied Biosystems, Foster City, CA, USA). PCR reagents were synthesized by Fermentas Life Sciences (Opelstrasse, Germany). The PCR mixture contained 100 ng genomic DNA, 0.25 µM of each primer, 0.2 mM dNTPs mixture, 1,5 à 2,0 mM Magnesium chloride and 0.04 unit of Taq DNA polymerase in a final reaction volume of 25 µl. PCRs were performed in a programmable thermalcycler with the following protocol: 94°C for 5 minutes; followed by 35 cycles of 94°C for 1 minute, desired primer annealing temperatures for 2 minutes and 72°C for 1 minute; with a final extension step of 72°C for 5 minutes. Amplicons of the different microsatellite markers was confirmed by running the PCR products on 2% agarose gel and visualizing under UV rays using gel documentation system (BIOMETRA Ti3, biomedizinische Analytik, GmbH, Gölttingen, Germany).
Following this, 15 μl of PCR products were loaded onto a 8% polyacrylamide gel with 50 bp ladder and electrophoreses were carried out in a double cassette plates. Gels were stained than were visualized and analyzed using Bio-Rad Gel Doc™XR.
-
Statistical Analysis
The program POPGENE (Version 1.32) was used to determine the polymorphism information content (PIC), the allele frequencies, number of alleles at each locus, effective number of alleles reflecting the interrelationship among different alleles, the population average heterozygosity (H) and the inbreeding coefficient (FIS).
We used Arlequin 3.5.1.2 (Excoffier et al., 2005) to determinate the FST values for pairwise comparisons of the breeds and their significance level for genetic differentiation, and to evaluate genetic structure of the populations. Parameters of genetic differentiation (F statistics), mean number of alleles across populations, observed, average expected (non-biased) and average observed heterozygosities were calculated using Genetix 4.05.2 software (Belkhir and Borsa 1998).
The program STRUCTURE (Pritchard et al., 2000) was used to analyze the genetic structure of our populations. This program infers the number of populations into which the analyzed genotypes can be divided. The program estimates the natural logarithm of the probability that a given genotype X is part of a given population K: ln Pr(X | K). This ensures that the groups are, as representatively as possible, samples from a single population. The program assumes that there are K populations with unknown gene frequency distribution at each locus pkl for the k = 1 . . . K populations and l = 1 . . . L loci contributing to the gene pool of the target population. Alleles at each locus are sampled independently for each individual, conditional on the proportion qi of its genotype in a given population. The program STRUCTURE uses the Markov Chain Monte Carlo method to separately estimate the posterior probability distribution of each parameter (particularly qi and qkl) in an integrated way over all the other parameters. This means that, in contrast to previous methodologies (Rannala and Mountain, 1997), it is not necessary to specify the gene frequencies of the contributing source populations in advance. We ran the program 12 times, fitting K from 1 to 10. All runs used a burn-in period of 100,000 iterations and a period of data collection of 100,000 iterations.
-
Results and discussion
-
Results
As a rule of thumb the FAO (1998) recommends to use only loci with a minimum number of four alleles for genetic distance studies. This was fulfilled for the eight chosen loci. The number of observed alleles per locus ranged from five alleles at locus OarFCB20 to 15 alleles at locus MAF214.
-
Genetic variation within populations
The parameters for the within-population genetic diversity are shown in Table 2. The studied population harboured 64 alleles with an average of 8 alleles per locus for 8 loci. Within the local goat populations, the average number of alleles (ANA) varied from 5.87 in Kebili locality goats to 7.62 for both of Nefzawa populations (Arbi Nefzawa and Serti Nefzawa). Number of effective alleles (Ne) varied from 3.84 in Degueche locality to 5.16 with Arbi Nefzawa population, and allelic richness (AR) varied from 2.13 in fatnassa locality to 5.23 with Serti Jerid population with an average of 5.16. The average expected heterozygosities lie in a narrow range of 0.72 to 0.8. Expected heterozygosity (He) of oases goat ecotypes varied from 0.725 in Souk Lahad locality to 0.803 with serti Jerid population with an overall mean value of 0.755. Mean estimate of observed heterozygosity (Ho) of overall oases ecotypes and loci was 0.658.
For the eight available markers, the greatest value of mean number of alleles (ANA), heterozygosities values (He and Ho) of and allelic richness (AR) was displayed by populations. In localities level, the locality of Bechni from Nefzawa region showed the greatest values for observed heterozygosity (Ho). The lowest value of these parameters was displayed in the two localities of El hamma and Degueche.
Deviations from Hardy–Weinberg equilibrium, after sequential Bonferroni correction, were significant for all loci population combinations (p < 0.01).
Table 2. Genetic diversity estimates for Tunisian goat populations
Population |
Sample size |
ANA |
Ne |
He±SD |
Ho±SD |
AR |
dEHW |
|||||||
Serti Jerid Arbi Jerid Serti Nefzawa Arbi Nefzawa Mean of populations Nafta Goat El hamma Goat Degueche Goat Tozeur Goat Kebili Goat Souk Lahad Goat Becnhi Goat Fatnassa Goat Mean of localities |
56 57 60 52 56.25 27 20 33 29 22 36 33 25 28.12 |
7.50 7.50 7.62 7.62 7.56 6.87 6.50 6.00 6.75 5.87 6.25 6.62 6.37 6.40 |
4.88±0.96 4.72±1.19 4.96±1.55 5.16±1.70 5.01±1.16 4.67±1.35 4.37±1.51 3.84±0.67 4.74±0.84 4.05±0.98 4.42±1.77 5.04±1.86 4.70±1.12 5.37±1.14 |
0.803±0.033 0.791±0.044 0.792±0.051 0.800±0.055 0.797±0.045 0.725±0.072 0.734±0.093 0.731±0.054 0.743±0.036 0.783±0.092 0.765±0.150 0.779±0.072 0.777±0.052 0.755±0.078 |
0.794±0.247 0.720±0.239 0.770±0.201 0.777±0.232 0.765±0.229 0.602±0.349 0.606±0.363 0.638±0.253 0.543±0.171 0.772±0.302 0.584±0.385 0.827±0.155 0.693±0.258 0.658±0.279 |
5.23 5.17 5.14 5.13 5.16 4.25 3.87 4.41 4.17 4.56 4.27 4.58 4.13 4.28 |
8 8 8 8 8 8 8 8 8 8 8 8 8 8 |
ANA, average number of alleles/Locus;
Ne, effective number of alleles;
AR, allelic richness;
He, expected heterozygosity; Ho, observed heterozygosity;
Fis, within-breed heterozygote deficiency;
dEHW, number of loci deviated from Hardy–Weinberg equilibrium for each population.
Parameters calculated with all markers
-
Genetic relationships and clustering
Fixation indices are shown in Table 3. FST values ranged from 0.025 to 0.128. The overall FST value was 0.058. Therefore roughly 6 % of genetic diversity can be explained by genetic variation between populations.
Mean value of inbreeding coefficient (FIS) was 0.139. This result allowed saying that the greater part of genetic diversity came from individual variations between animals.
Nm can be interpreted as the effective number of migrants exchanged between demes/subpopulations per generation (Wright 1969). If Nm increases, FST decreases, and vice versa. In general, Nm < 1 results in relatively large values for FST. Wright interpreted Nm as a virtual number of migrants. But that Nm >> 1 results in little divergence (i.e., low FST). However, low migration results in a large FST.
In this study, we obtained proportional important Nm values varied from 1.70 to 9.75 with an overall value of 4.06 which predict little genetic divergence in the population.
The topology of trees based on Nei’s minimum and Reynolds’ distances was similar. Therefore only trees based on Nei’s minimum distances are presented in Figures 4 and 5.
Table 3. Fixation indices (FIS, FIT FST) and Nm according to Weir & Cockerham (1984)
Primer |
FIS |
FIT |
FST |
Nm |
||||
ILSTS087 |
0.104±0.118 |
0.224±0.152 |
0.128±0.058 |
1,70 |
||||
TGLA057 |
0.510±0.068 |
0.528±0.073 |
0.033±0.022 |
7,33 |
||||
McM527 |
-0.069±0.071 |
-0.022±0.078 |
0.044±0.014 |
5,43 |
||||
RM004 |
0.514±0.186 |
0.569±0.165 |
0.112±0.028 |
1,98 |
||||
OarFCB20 |
0.182±0.027 |
0.203±0.028 |
0.025±0.020 |
9,75 |
||||
MAF214 |
0.222±0.072 |
0.250±0.062 |
0.037±0.016 |
6,51 |
||||
MAF065 |
-0.295±0.026 |
-0.242±0.026 |
0.041±0.021 |
5,85 |
||||
CSSM31 |
-0.064±0.058 |
-0.017±0.048 |
0.044±0.026 |
5,43 |
||||
Mean |
0.139±0.012 |
0.189±0.054 |
0.058±0.063 |
4,06 |
Nm: Gene flow estimated from FST, Nm = 0.25(1 - FST)/ FST.
|
Figure 4. Unweighted pair group method with arithmetic mean-distance tree based on an average Nei’s minimum distance from 100 Bootstrap-samples with 50 individuals within each population. |
|
Figure 5. Unweighted pair group method with arithmetic mean-distance tree based on Nei’s minimum distance between the eight studied localities. PHYLIP Version 3.5. |
Network tree based on Nei’s minimum distance values is shown in Figure 4. The clustering showed the separation of each local population. Each population formed their own separate cluster.
Tree based on the distance between animals in same locality showed that goat populations are not grouped according to their geographic origin (Jerid or Nefzawa). Goats from the different ecotypes or locality were mixed together.
Two STRUCTURE analyses were carried out in this study. The first was performed using the separate goat populations and the different localities; the second was performed using only the local goat populations. The analyzed data have a complex underlying genetic structure.
The program STRUCTURE was first run with a number of expected populations, ranging from K = 1 to K = 10, so as to choose the appropriate value of K to better model the whole data set. The most probable number of clusters was estimated according to the best mean log-likelihood score resulting in the highest percentage of membership coefficient (q) to each cluster (Pritchard et al. 2000). The membership patterns of each of the four current goat populations in each of the eight inferred clusters were substantially the same as that reported for the complete data set. The best value of ln Pr(X | K) was obtained for K=8 (-5303.7). These eight inferred populations would correspond to the “geographical” populations from which our current populations were derived.
The program STRUCTURE computes the allelic frequencies expected in each locus for the inferred populations and, interestingly enough, the proportion of the four current goat populations in each of the eight inferred populations (Table 4). Each inferred population was at least formed two of the current populations.The first inferred population were basically formed by Arbi Jerid and Serti Nefzawa individuals. The second and the fourth inferred populations were basicly formed by participation of the three populations Serti Jerid, Arbi Nefzawa and Serti Nefzawa showing that these breeds had a particular genetic background that is possible to differentiate. The same thing was noticed with the third and the seventh inferred clusters and the fifth and the sixth inferred population. The fifth and sixth inferred clusters were basically formed by both Jerid populations. The eighth inferred population characterizes the Serti populations in the two geographical clusters (Nefzawa and Jerid).
Table 4. Proportion of membership of each of the four current goat populations in each of eight inferred clusters using the program STRUCTURE
Source populations |
Inferred populations |
||||||||
1 |
2 |
3 |
4 |
5 |
6 |
7 |
8 |
||
Arbi Jerid |
0.139 |
0.070 |
0.149 |
0.093 |
0.137 |
0.127 |
0.111 |
0.174 |
|
Serti Jerid |
0.097 |
0.169 |
0.070 |
0.188 |
0.200 |
0.142 |
0.052 |
0.082 |
|
Arbi Nefzawa |
0.096 |
0.154 |
0.174 |
0.134 |
0.075 |
0.098 |
0.142 |
0.126 |
|
Serti Nefzawa |
0.132 |
0.139 |
0.145 |
0.177 |
0.068 |
0.105 |
0.164 |
0.070 |
|
FST for inferred populations |
0.185 |
0.136 |
0.169 |
0.163 |
0.198 |
0.171 |
0.195 |
0.179 |
-
Discussion
-
Genetic diversity of Tunisian goat
-
All microsatellite loci exhibited more than 4 alleles indicating they were appropriate for a genetic diversity analysis (Barker 1994). Furthermore, Takezaki & Nei (1996) have determined that gene diversity should be in the range of 0.3–0.8 in the populations, in order that markers to be useful for measuring genetic variation. Average value of gene diversity (He) for all populations in the present study was 0.79. This confirmed that these markers were appropriate for measuring genetic variation. Similar results (0.747) were recently reported with Singh et al. (2015) studying the gaddi goat breed of Western Himalayas, also (GX., 2015) found values of mean expected heterozygosity varied from 0.25 to 0.78 in his study genetic variability of ten Chinese indigenous goats using MHC-linked microsatellite markers and with Okpeku et al. (2011) in his analysis of microsatellite-based genetic diversity of goats in southern Nigeria. These values of mean heterozygosity were very close to 0.70 across all 25 microsatellite loci in six Portuguese goat breeds (Bruno-de-Sousa et al., 2011), 0.72 for Egyptian goats (Agha et al., 2008), 0.63–0.69 in South African goats (Visser et al., 2004), 0.68 in the Brown Short haired goat in the Czech Republic (Jandurova et al., 2004), 0.611–0.84 for Chinese goats (Qi et al., 2009) and 0.61–0.77 in Spanish Guadarrama goats (Serrano et al., 2009).The Tunisian local goats have value mean heterozygosity quiet close to 0.69 of a large group of goat breeds from Europe and the Near East (Cañon et al., 2006).
Allelic richness in Tunisian goats had an average of 3.87 to 5.23 is lower than Indian goats (8.1–9.7) by Rout et al. (2008), the average of 7.3 in Iranian goats (Mahmoudi et al., 2010), the average of 5.9 in Canary Island goats (Martínez et al., 2006), and values varied from 7.61 to 8.19 of domestic goat of Albania (Hoda et al., 2011).
The mean number of alleles was relatively the same in localities and in populations, indicating high level of genetic variability of local Tunisian goats in both localities and population’s levels in this study.
-
Genetic differentiation according to geography and populations
The FST values ranged from 0.025for OarFCB20 to 0.128 for ILSTS087. Low FST indicates some measure of gene flow between the sampled populations, with ILSTS087 locus recording the highest gene flow of 21.95. Mujibi (2005) reported same FST value of 5.8% for WAD goats in Kenya; therefore, gene flow estimates in this study (values varied from 8.19 to 21.95 with an overall value of 13.65) suggest mobility and considerable exchange of genetic material among these goats. These could be attributed to the fact that some of these animals originate from the other region. Because of the low distance between Jerid and Nefzawa regions and the direct access to animals the gene flow was high in all markers.
Genetic distance measures based on gene frequencies can be a good indicator of the history of the goat and spatial distribution and origin.
On the contrary, the low genetic diversity between ecotypes is certainly due to the mode of reproduction, the extensive system of management of farms and movement of animals with their owners/ shepherd of an area to another. Usually, the farmer buys goats in the market, or from neighbour farms without any information or control of their origin. These factors favour a permanent gene flow and create greater diversity within population. The pattern of clustering observed with the allele-sharing distance measures among individual goats reflected the admixture of individuals coming from different ecotypes. This reflects also the high gene flow and intermixing of gene pools between ecotypes and suggests that they are genetically very close Mahammi et al. (2015).
Results obtained using the program STRUCTURE suggested that the studied populations had large cryptic structures. Theoretically, this structure would be a consequence of the genetic background of the original populations from which present-day breeds were derived. However, the inferred populations do not necessarily correspond to “real” ancestral populations, and they can be determined simply by the sampling scheme (Pritchard et al., 2000). It seems to be quite difficult to estimate the allelic frequencies of the “original” populations when populations are not well differentiated, and a large proportion of individuals have some degree of admixture. The genetic structure observed here could be a consequence of both the absorption of individuals from other populations and reproductive isolation producing bottlenecks at a population level. Both aspects could affect the present results. Very close results were reported by (Álvarez et al. 2004) when he studied the genetic admixture of six sheep breeds in northern Spain.
-
Conclusion
In comparison with previous results of genetic diversity at phenotypic and biochemical levels in Tunisian oases goats (Nafti et al., 2014 and Nafti et al., 2013), our obtained results confirm the high amount of genetic variability that exist. The use of classical parameters, such as F-statistics, to assess genetic differentiation and gene flow assumes the great introgression of genes in the studied populations into each other. Population structure results in a low level of genetic differentiation due to uncontrolled mating systems and breeder’s management. The obtained results of genetic differentiation were confirmed at population’s level such as localities level. However, Tunisian oases local goat populations should be safeguarded as an important reservoir of genetic diversity.
Acknowledgements
The authors thank Professor Ben Naceur M’barek, General Director of National Gene Bank of Tunisia for his permission to perform the main part of analyzes in the national gene bank and its contribution while working in the laboratory.
-
References
Agha SH, Pilla F, Galal S, Shaat I, D’Andrea M, Reale S, Abdelsalam A Z , Li M H (2008) Genetic diversity in Egyptian and Italian goat breeds measured with microsatellite polymorphism. J. Anim. Breed. Genet., 125(3): 194–200
Álvarez I, Royo L J, Fernández I, Gutiérrez J P, Gómez E, Goyache F (2004) Genetic relationships and admixture among sheep breeds from Northern Spain assessed using microsatellites. J. Anim. Sci.. 82:2246–2252
Barker J (1994) A global protocol for determining genetic distances among domestic livestock breeds, Proc. 5th World Congr, Genet. Appl. Livest. Prod., 21, 501–508
Belkhir K, Borsa P, Chikhi L, Raufaste N, Bonhomme F(2002) GENETIX, Version 4.05 logiciel sous Windows TM pour la Genetique des populations. Laboratoire Génome, Populations, Interactions CNRS UMR 5000. Universite´ Montpellier II, Montpellier, France
Cañon J, García D, García-Atance M A, Obexer-Ruff G, Lenstra J A, Ajmone-Marsan P, Dunner S, Econogene Consortium (2006) Geographical partitioning of goat diversity in Europe and the Middle East. Anim. Genet., 37(4): 327–34.
Excoffier L, Laval G, Schneider S (2005) Arlequin ver. 3.0: An integrated software package for population genetics data analysis. Evolutionary Bioinformatics Online 1:47-50
Food and Agriculture Organization of the United Nations FAO (1998) Secondary Guidelines for Development of National Farm Animal Genetic Resources Management Plans. Measurement of Domestic Animal Diversity (MoDAD): Original Working Group Report. Home page address: http://dad.fao.org/en/refer/library/guideline/workgrp.pdf
Hoda A, Hyka G, Dunner S, Obexer-Ruff G, Econogene Consortium (2011) Genetic diversity of albanian goat breeds based on microsatellite markers. Arch. Zootec. 60 (231): 607-615
Jandurova OM, Kott T, Kottova B, Czernekova V (2004) Seven microsatellite markers useful for determining genetic variability in white and brown short-haired goat breeds. Small Ruminant Res. 52: 271–274
Mahammi F Z, Gaouar S B S, Laloe D, Faugeras R, Tabet-Aoul N, Rognon X, Tixier-Boichard M, Saidi-Mehtar, N (2015) A molecular analysis of the patterns of genetic diversity in local chickens from western Algeria in comparison with commercial lines and wild jungle fowls, J. Anim. Breed. Genet. 1–12. © Blackwell Verlag GmbH
Mahmoudi B, Bayat M, Sadeghi R, Babayev M, Abdollahi H (2010) Genetic diversity among three goat populations assessed by microsatellite DNA markers in Iran. Global Vet., 4(2): 118–124
Martínez AM, Acosta J, Vega-Pla JL, Delgado JV (2006) Analysis of the genetic structure of the canary goat populations using microsatellites, Livestock Sci., 102: 140–145
Mujibi NF (2005) Genetic characterization of West African Dwarf (WAD) goats using microsatellite markers. Kenyatta University, Nairobi, Kenya. (MSc Thesis)
Nafti M, Khaldi Z, Haddad B (2014) Multivariate characterization of morphological traits in local Tunisian oases goats, Animal Genetic Resources, 55, 29–38. © Food and Agriculture Organization of the United Nations, doi:10.1017/S2078633614000265
Nafti M, Khaldi Z, Haddad B (2013) Protein polymorphism of goat of goats in Tunisian Oases, BIOMIRROR: 5-12 / bm-1422800113
Nei M (1987) Molecular Evolutionary Genetics, Columbia University press, New York, USA.
Okpeku M, Peters S O, Ozoje M O, Adebambo O A, Agaviezor B O, O’Neill M J,
Imumorin IG (2011) Preliminary analysis of microsatellite-based genetic diversity of goats in southern Nigeria. Animal Genetic Resources, 49, 33–41. © Food and Agriculture Organization of the United Nations
Pritchard J K, Stephens M, Donnelly P (2000) Inference of population structure using multilocus genotype data, Genetics 155:945–959
Qi Y, Luo J, Han X-F, Zhu Y-Z, Chen Ca, Liu J-X, Sheng H-J (2009) Genetic diversity and relationships of 10 Chinese goat breeds in the Middle and Western China. Small Ruminant Res., 82: 88–93
Rannala B, Mountain J L (1997) Detecting immigration by using multilocus genotypes. Proc. Natl, Acad. Sci. U.S.A. 94:9197–9221
Rout P K, Joshi M B, Mandal A, Laloe D, Singh L, Thangaraj K (2008) Microsatellite- based phylogeny of Indian domestic goats. BMC Genet., 9: 11
Serrano M, Calvo J H, Martínez M, Marcos-Carcavilla A, Cuevas J, González C, Jurado J J, de Tejada P D (2009) Microsatellite based genetic diversity and population structure of the endangered Spanish Guadarrama goat breed. BMC Genet., 29: 61
Singh G, Thakur Y, Kour A, Sankhyan V, Katoch S (2015) Genetic characterization of Gaddi goat breed of Western Himalayas using microsatellite markers, Veterinary World 8(4); 527-531
Takezaki N, NeiM (1996) Genetic distances and reconstruction of phylogenetic trees from microsatellite DNA. Genetics, 144, 389–399
Visser C, Hefer C A, Van Marle-Köster E, Kotze A (2004) Genetic variation of three commercial and three indigenous goat populations in South Africa. South African J. Anim. Sci., 34(Suppl. 1): 24–27
Wright S (1969) Evolution and the Genetics of Populations: The Theory of Gene Frequencies. The University of Chicago Press, Chicago, Illinois