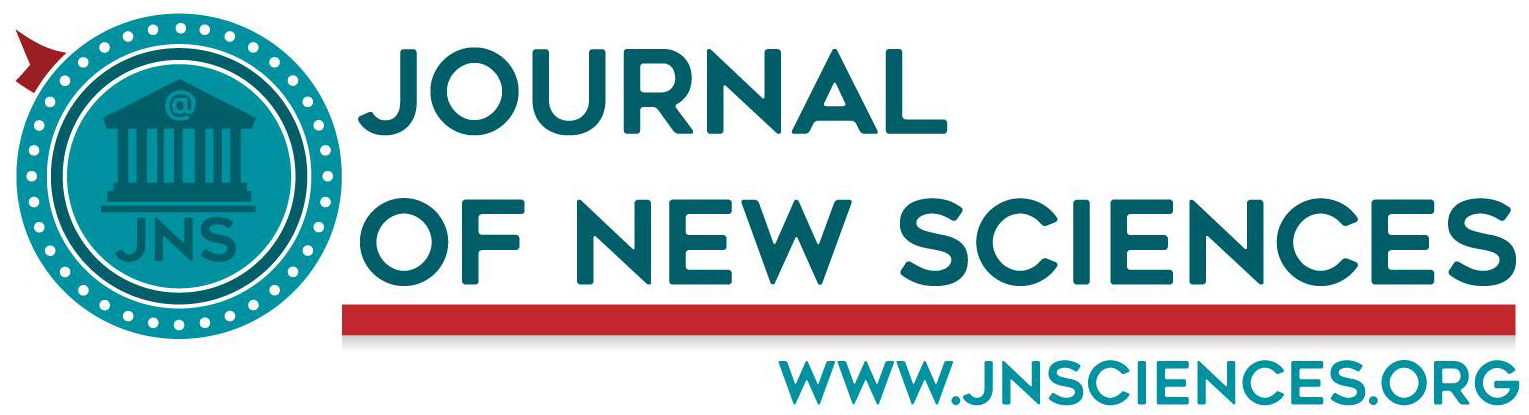
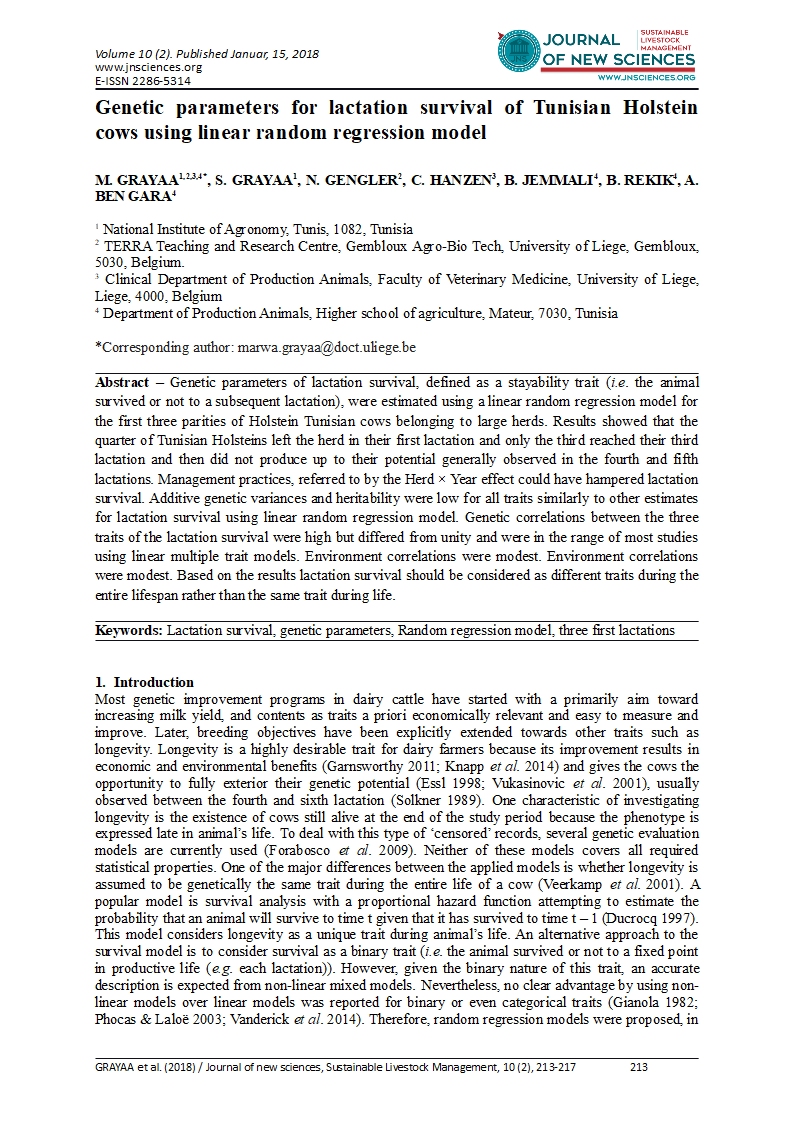
- Category: Volume 10
- Hits: 5145
Genetic parameters for lactation survival of Tunisian Holstein cows using linear random regression model
M. GRAYAA1,2,3,4
S. GRAYAA1
N. GENGLER2
C. HANZEN3
B. JEMMALI4
B. REKIK4
A. BEN GARA4
1 National Institute of Agronomy, Tunis, 1082, Tunisia
2 TERRA Teaching and Research Centre, Gembloux Agro-Bio Tech, University of Liege, Gembloux, 5030, Belgium.
3 Clinical Department of Production Animals, Faculty of Veterinary Medicine, University of Liege, Liege, 4000, Belgium
4 Department of Production Animals, Higher school of agriculture, Mateur, 7030, Tunisia
Abstract – Genetic parameters of lactation survival, defined as a stayability trait (i.e. the animal survived or not to a subsequent lactation), were estimated using a linear random regression model for the first three parities of Holstein Tunisian cows belonging to large herds. Results showed that the quarter of Tunisian Holsteins left the herd in their first lactation and only the third reached their third lactation and then did not produce up to their potential generally observed in the fourth and fifth lactations. Management practices, referred to by the Herd × Year effect could have hampered lactation survival. Additive genetic variances and heritability were low for all traits similarly to other estimates for lactation survival using linear random regression model. Genetic correlations between the three traits of the lactation survival were high but differed from unity and were in the range of most studies using linear multiple trait models. Environment correlations were modest. Environment correlations were modest. Based on the results lactation survival should be considered as different traits during the entire lifespan rather than the same trait during life.
Keywords: Lactation survival, genetic parameters, Random regression model, three first lactations
1. Introduction
Most genetic improvement programs in dairy cattle have started with a primarily aim toward increasing milk yield, and contents as traits a priori economically relevant and easy to measure and improve. Later, breeding objectives have been explicitly extended towards other traits such as longevity. Longevity is a highly desirable trait for dairy farmers because its improvement results in economic and environmental benefits (Garnsworthy 2011; Knapp et al. 2014) and gives the cows the opportunity to fully exterior their genetic potential (Essl 1998; Vukasinovic et al. 2001), usually observed between the fourth and sixth lactation (Solkner 1989). One characteristic of investigating longevity is the existence of cows still alive at the end of the study period because the phenotype is expressed late in animal’s life. To deal with this type of ‘censored’ records, several genetic evaluation models are currently used (Forabosco et al. 2009). Neither of these models covers all required statistical properties. One of the major differences between the applied models is whether longevity is assumed to be genetically the same trait during the entire life of a cow (Veerkamp et al. 2001). A popular model is survival analysis with a proportional hazard function attempting to estimate the probability that an animal will survive to time t given that it has survived to time t – 1 (Ducrocq 1997). This model considers longevity as a unique trait during animal’s life. An alternative approach to the survival model is to consider survival as a binary trait (i.e. the animal survived or not to a fixed point in productive life (e.g. each lactation)). However, given the binary nature of this trait, an accurate description is expected from non-linear mixed models. Nevertheless, no clear advantage by using non-linear models over linear models was reported for binary or even categorical traits (Gianola 1982; Phocas & Laloë 2003; Vanderick et al. 2014). Therefore, random regression models were proposed, in a linear context, for modelling survival as a different trait across lactations (Jairath et al. 1998; Veerkamp et al. 2001). The purposes of this study were to estimate genetic parameters of lactation survival in the first three lactations using a linear random regression model.
2. Material et Methods
2.1. Data
Pedigree and data regarding survival of Tunisian Holstein cows (n= 25,981) that participated in the official milk recording in the period from 2000 to 2014, were provided by the Tunisian Genetic Improvement Center (Tunisian Livestock and Pasture Office). The animals belonged to herds of at least 50 cows in order to evaluate informative herds with good herd management (van Pelt et al. 2016). Several editing criteria were employed to ensure data’s quality: Plausible upper and lower limits for age at first calving were set at > 20 or < 42 months.
Lactation survival: The observed trait was defined as cow’s survival to the subsequent lactation. Cows that have not subsequent lactation were non-survivor cows and were coded as 1. Cows that calved back were survivor cows and were coded 2. Second lactation cows had necessarily a first lactation. Third lactation cows had necessarily a first and a second lactation. Since records used in this study were taken until the end of 2014, and the occurrence of a following calving was checked up to 21th October 2017, we considered that cows had sufficient opportunity to calve again. Cows were sired by 832 sires whose 22.5% have more than 30 daughters.
2.2. Model
One three-trait model (survival in the three first lactations) analysis was run using a linear random regression model. The model was written under matrix notation as follows:
(1)
where y is a vector of survival in the first, second and third lactation; b is a vector of fixed effects of herd × year of calving, age of calving, a and p are vectors of random regression coefficients for additive genetic and permanent environment effects, respectively; Qis a matrix for for the null-order Legendre polynomial (constant= 1); X, Z are incidence matrices linking observations with respective effects, and e is a vector of residuals.
The covariance matrix structure of the model is as follows:
, (2)
Where is a 3×3 (co)variance matrix of the additive genetic random regression coefficients;
is the additive relationship matrix among all animals;
is the 3×3 (co)variance matrix of the random permanent environment regression coefficients, and
is a 3×3 diagonal matrix of residual variance. For all traits, additive genetic variance (σa2), permanent environment variance (σp2), residual variance (σe2), phenotypic variance (σT2) were obtained by the appropriate functions of regression coefficients Ka andKp.
Heritability(h2) estimatesof survival were defined as the ratio of σa2 to σT2. Residual variances were assumed to be independent and constant across lactations. To avoid environmental covariances being considered as genetic covariances, within animal, survival was modeled by a permanent environment effect, as proposed by Bastin et al (2012). Variance components were estimated using REMLF90 software (Misztal et al. 2002).
3. Results and discussion
The number of survivor and culled cows in each lactation and their proportions compared to the initial number of cows are displayed in Table 1. Results showed a shorter than expected stay of Holstein cows in Tunisian large herds. Indeed, from studied cows, the quarter left the herd in their first lactation, only 53% of them reached their second lactation and only the third reached their third lactation. It was reported that Holsteins produce their maximum milk yield in the fourth and fifth lactations in their countries of origin (Solkner 1989). Unfortunately, Tunisian cows were culled much earlier. Many reasons may have contributed to compromise the lactation survival (i.e. Herd management, climatic conditions, food shortage, compromised reproduction or production performances). Thus, regarding the fact that only Herd× Calving year and age of calving effects were modelled within the fixed effects in the current study, other effects are hypothetical and will not be discussed since they are not included in the model. Herd could be considered as an effect that account of management practices and other environmental conditions that are possible to vary from herd to herd. The interaction Herd× Calving year makes it possible to take account of the difference related to the system in control of breeding and in particular to the food (Bouallegue et al. 2014). Thus, poor management could have hampered lactation survival in Tunisian large herds.
Table 1. Survivor and culled cows in each lactation and their proportions compared to the initial number of cows |
||||||||
Code |
First parity |
|
Second parity |
|
Third parity |
|||
|
Number |
% |
|
Number |
% |
|
Number |
% |
Cows coded 1 |
6443 |
24.8 |
|
5754 |
22.1 |
|
4460 |
17.2 |
Cows coded 2 |
19538 |
75.2 |
|
13784 |
53.0 |
|
9324 |
35.9 |
Heritability and variance components (σa2, σp2, σe2and σT2) are shown in Table 2. Additive genetic variances of lactation survival were low similarly to other estimates for lactation survival using linear random regression model (Gengler et al. 2005). Heritability estimates for lactation survival decreased over lactation. An explanation could be the reduction of the cows surviving to successive lactations and then reduction of the data. Estimates of heritability were lower than most estimates from survival analysis that ranged from 0.05 to 0.181 (M’hamdi et al. 2010) but were in the range of most estimates from linear random regression models that ranged from 0.016 to 0.110. Nevertheless, heritability estimates from linear models are not directly comparable to estimates from survival models (Forabosco et al. 2009).
Table 2. Heritability (h2) estimates, additive genetic variances (σa2), permanent environment variance (σp2), residual variances, and total variance (σT2) for lactation survival |
|||||
Trait |
σa2 (10-2) |
σp2(10-4) |
σe2 |
σT2 |
h2 |
Survival in the first parity |
0. 45 |
0.50 |
0. 17 |
0.17 |
0.03 |
Survival in the second parity |
0. 37 |
0.53 |
0.18 |
0.18 |
0.02 |
Survival in the third parity |
0. 50 |
1.3 |
0.20 |
0.20 |
0.02 |
Genetic and environmental correlations between survivals to different lactations are shown in Table 3. Genetic correlations between the three traits of the lactation survival were high but differed from unity. Environmental correlations between survival traits were modest. The higher is the distance between parities, the lower are the correlations estimates. These results support those of most studies using linear multiple-trait models. Indeed, it was reported that genetic correlations between parities significantly differ from unity and ranged from 0.33 to 0.96. The correlations estimates decreased with increasing distance between parities (Visscher & Goddard 1995; Jairath et al. 1998; Boettcher et al. 1999; Veerkamp et al. 2001; Sewalem et al. 2007; Holtsmark et al. 2009; van Pelt & Veerkamp 2014). Results suggest that survival is genetically and environmentally not the same trait during the entire life, because genetic correlations differ from unity between different parities and environment correlations were modest.
Table3. Genetic (below the diagonal) and permanent environment correlations between survival in first, second and third lactations |
|||
Survival trait |
First lactation |
Second lactation |
Third lactation |
First lactation |
1.00 |
0. 71 |
0.47 |
Second lactation |
0.97 |
1.00 |
0.60 |
Third lactation |
0.95 |
0.96 |
1.00 |
4. Conclusion
For this investigation, the data was, a priori, limited to large herds that represented a little proportion of all herd-book breeders registered farms in the Holstein Cattle but representing an important proportion of cows. Heritability estimates were low but similar to previous literature estimates from studies that used a comparable model. Based on these results, further studies aiming to investigate the possible indirect selection on causes of culling (e.g. production traits) would be interesting.
Author contribution
Marwa Grayaa edited the data, developed the model and prepared the manuscript with contributions from all co-authors.
Aknowlegement
Authors are thankful to the Genetic Improvement Center (Tunisian Livestock and Pasture Office) for providing the data. Computational resources have been provided by the Consortium des Équipements de Calcul Intensif (CÉCI) funded by the National Fund for Scientific Research (F.R.S - FNRS, Brussels, Belgium) under Grant No. 2.5020.11.
5. References
Bastin C, Berry DP, Soyeurt H, Gengler N (2012) Genetic correlations of days open with production traits and contents in milk of major fatty acids predicted by mid-infrared spectrometry. J Dairy Sci 95: 6113–6121.
Boettcher PJ, Jairath LK , Dekkers JCW (1999) Comparison of methods for genetic evaluation of sires for survival of their daughters in the first three lactations. J Dairy Sci 82:1034–1044.
Bouallegue M, M’hamdi N, Ben Hamouda M, Haddad B (2014) Study of non-genetic factors on the shape of lactation curves for milk yield, fat and protein percents of Holstein-Friesian cows under hot Mediterranean climate. Archiva zootechnica 17: 55-75.
Ducrocq V, Quaas RL, Pollak EJ (1987) Length of productive life of dairy cows :justification of weirbull model. J dairy Sci 71: 3061–3070.
Brotherstone SR, Veerkamp F, Hill WG (1997) Genetic parameters for a simple predictor of the lifespan of Holstein-Frie- sian dairy cattle and its relationship to production. J Anim Sci 65:31–37.
Essl A (1998) Longevity in dairy cattle breeding: a review. Livest Prod Sci 57: 79–89.
Forabosco F, Jakobsen JH, Fikse WF (2009) International genetic evaluation for direct longevity in dairy bulls. J Dairy Sci 92: 2338–2347.
Garnsworthy P (2011) The environmental impact of fertility in dairy cows. Western Canadian Dairy Seminar 23: 181–190.
Gengler N, Vanderick S, Mayeres P, Gillon, A, Croquet C (2005) Genetic evaluation of cow survival using a lactation random regression model. Interbull Bulletin 33: 176–180.
Gianola D (1982) Theory and analysis of threshold characters. J Anim Sci 54: 1079–1096.
Holtsmark M, Heringstad B, Ødegård J (2009) Predictive abilitties of different statistical models for analysis of survival data in dairy cattle. J Dairy Sci 92:5730–5738.
Jairath L, Dekkers J C M, Schaeffer LR, Liu Z, Burnside EB, Kolstad B (1998) Genetic evaluation for herd life in Canada, J Dairy Sci 81: 550–562.
Knapp JR, Laur GL, Vadas PA, Weiss WP, Tricarico JM (2014) Enteric methane in dairy cattle production: quantifying the opportunities and impact of reducing emissions. J Dairy Sci 97: 3231–3261.
Matos C A, Thomas DL, Gianola D, Perez-Enciso M, Young LD (1997) Genetic analysis of discrete reproductive traits in sheep using linear and non-linear models: II Goodness of fit and predictive ability. J Anim Sci 75: 88–94.
M’hamdi N, Aloulou R, Bouallegue M, Brar SK, Ben Hamouda M (2010) Study on functional longevity of Tunisian Holstein dairy cattle using a Weibull proportional hazard model. Livest Science 132: 173–176.
Misztal I, Tsuruta S, Strabel T, Auvray B, Druet T, Lee DH (2002) BLUPF90 and related programs (BGF90). In: Proceedings of the 7th World Congress on Genet Applied to Livestock Production. Montpellier, France.
Phocas F and Laloë D (2003) Evaluation models and genetic parameters for calving difficulty in beef cattle. J Anim Sci 81: 933–938.
Sewalem A, Miglior F, Kistemaker GJ, Sullivan P, Huapaya G, Van Doormaal B J (2007) Short communication: Modification of genetic evaluation of herd life from a three-trait to a five- trait model in Canadian dairy cattle. J Dairy Sci 90:2025–2028.
Solkner J (1989) Genetic relationships betwee n level of production in different lactations , rate of maturity and longevi ty in a dual purpose cattle population. Livest Prod Sci 23:33–45.
Van Pelt ML, Meuwissen THE, De Jong G, Veerkamp RF (2014) Genetic analysis of longevity in Dutch dairy cattle using random regression. J Dairy Sci98:4117–4130.
Van Pelt ML, Dejong G, Veerkamp RF: Changes in the genetic level and the effects of age at first calving and milk production on survival during the first lactation over the last 25 years. Animal 10: 2043–2050, 2016.
Vanderick S, Troch T, Gillon A,Glorieux G, Gengler N (2014) Genetic parameters for direct and maternal calving ease in Walloon dairy cattle based on linear and threshold models. J Anim Breed Genet 131: 513–21.
Veerkamp R F, Brotherstone S, Engel B,Meuwissen T H E (2001) Analysis of censored survival data using random regression models. Anim Sci 72:1–10.
Vukasinovic N, Moll J, Casanova L (2001) Implementation of a routine genetic evaluation for longevity based on survival analysis techniques in dairy cattle populations in Switzerland. J dairy sci 84:2073–2080.
Visscher PM, Goddard ME(1995) Genetic parameters for milk yield, survival, workability, and type traits for Australian dairy cattle. J Dairy Sci 78:205–220.